CIRCLE Group
We have multliple PhD and PostDoc positions available. Feel free to e-mail me your CV and ideas. Below are the members of the CIRCLE group.

Simone Foti is a Postdoctoral Research Associate in the Department of Computing at Imperial College London. Previously, he obtained a PhD at the University College London (UCL) by researching “Latent Disentanglement for the Analysis and Generation of Digital Human Shapes”, which found applications in computer vision, graphics and plastic surgery. During the PhD, he gained industry experience as a Research Scientist Intern at Disney Research Studios (Zurich) and Adobe Research (Paris). Simone also obtained an MRes in Medical Imaging from UCL, an MSc in Biomedical Engineering at Politecnico di Milano, and a BSc in Computer Science at the University of Trieste. Currently, his research interest lies at the intersection of geometric deep learning, computer graphics, and computer vision. Through his research, Simone strives to understand how to solve problems in non-Euclidean domains such as graphs and meshes. He is currently working on multiple projects ranging from shape and texture generation, latent disentanglement, mesh sampling, and 3D reconstruction. More information here.
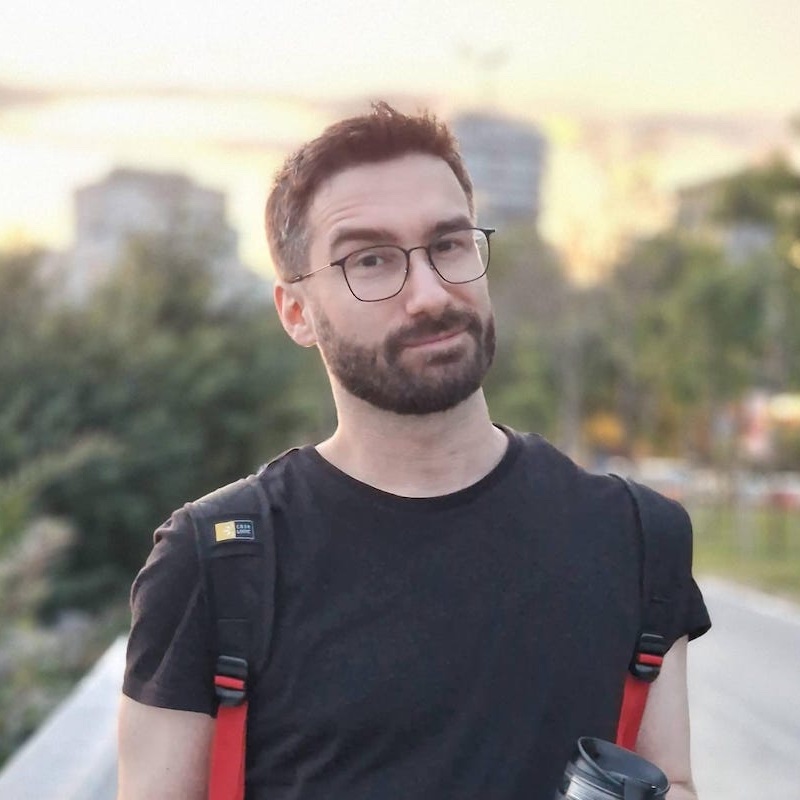
Melih Barsbey is a Postdoctoral Researcher. Melih’s research focuses on examining the synergy and trade-offs between compressibility and robustness in machine learning models, and training decisions which allow these to be obtained simultaneously. His past research also includes publications on human-AI complementarity, graphical latent variable models, and tensor factorization methods. Melih holds a PhD in Computer Engineering, BA and MA in Psychology from Boğaziçi University, Istanbul.
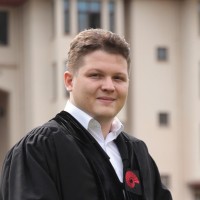
Caner Korkmaz is a PhD student in the Department of Computing at Imperial College London in the CIRCLE Group. Previously, he obtained an MPhil in Advanced Computer Science from the University of Cambridge with the research thesis “Stable 3D Diffusion: Text-to-3D Shape using Latent Point Diffusion Models”. Before that, he obtained Computer Engineering and Mathematics degrees at Koç University. During these, he obtained industry experience in applying deep learning to the construction and medical healthcare sectors. His research interests focus on computer vision, generative deep learning, and topological data analysis in/with deep learning and theoretical aspects of deep learning, currently focused on the generalization gap of neural networks and integration of topological data analysis into deep learning.
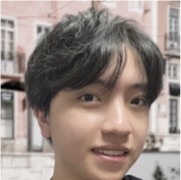
Zhengdi Yu is a PhD student in the CIRCLE Group at the Department of Computing, Imperial College London. Prior to this, he obtained an MRes in Computer Science from Durham University. He also gained industry experience as a research intern at Tencent AI Lab. His research interests primarily focus on 3D computer vision, with a particular emphasis on 4D motion representations of articulated bodies, such as hands and human bodies, as well as parametric models. He also has experience in 3D shape reconstruction and scene understanding towards applications for real-world problems.
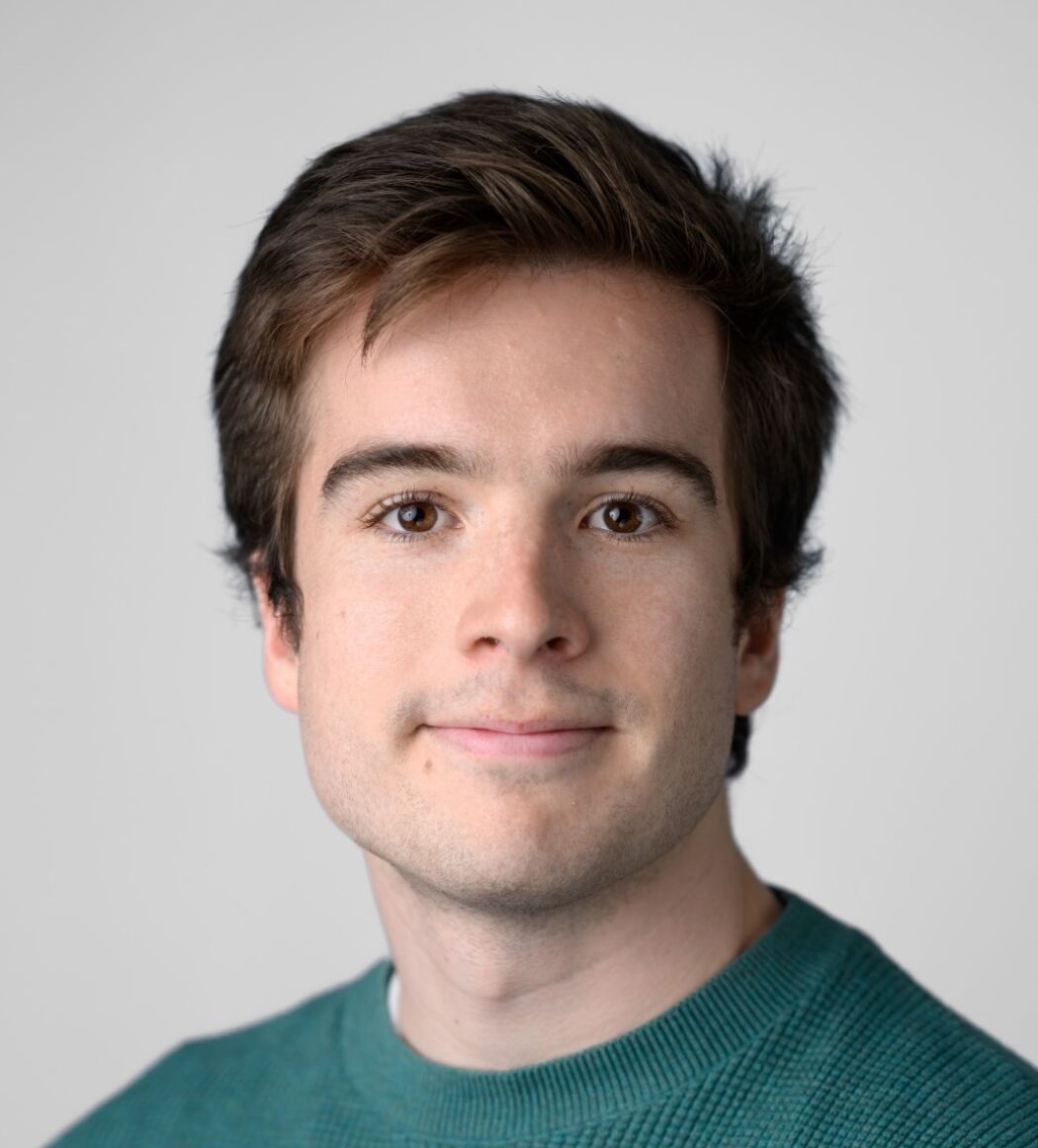
Lucas Prieto is currently a PhD student at Imperial College London funded by the Safe and Trusted AI - Center for Doctoral Training. He obtained a Bsc in Computing Science and Mathematics from the University of Glasgow and an Msc in Data Science from the University of Amsterdam. He also worked in industry for a year as a Research Data Scientist at SocialDatabase. His current foci of interests include exploring interpretable features and structures in deep learning models and their relationship to compression and generalisation.
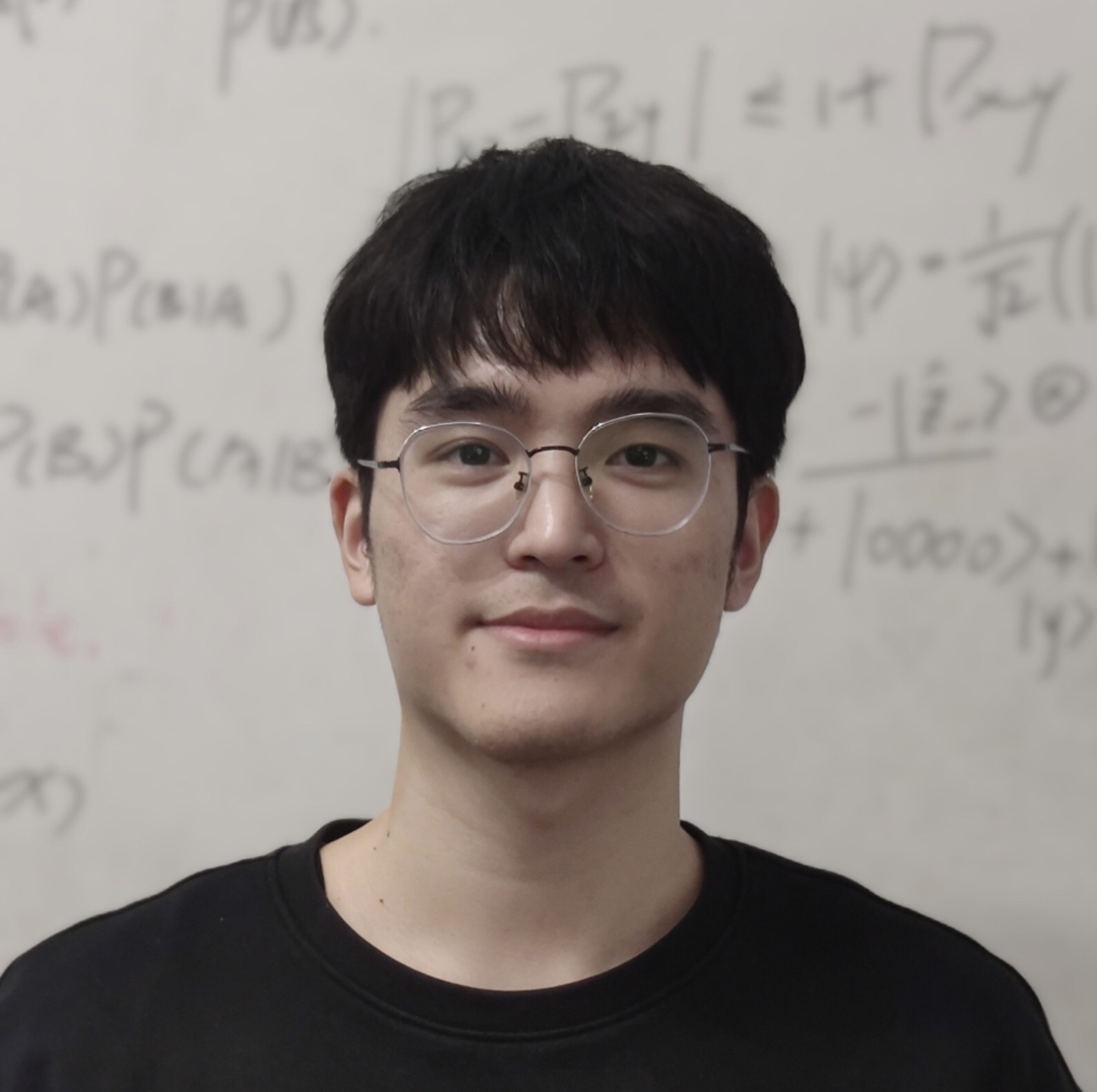
Yiming Huang is a PhD student and Lee Family scholar at the Department of Computing, Imperial College London. Previously, he obtained an MSc in Computer Science from the University of Electronic Science and Technology of China (UESTC). His research interests primarily focus on developing novel topological deep learning methods for understanding complex and higher-order graph structures. His broader interests also include generative models, graph representation learning, higher-order network analysis, and their interdisciplinary applications. More information here.